How to level a wood floor
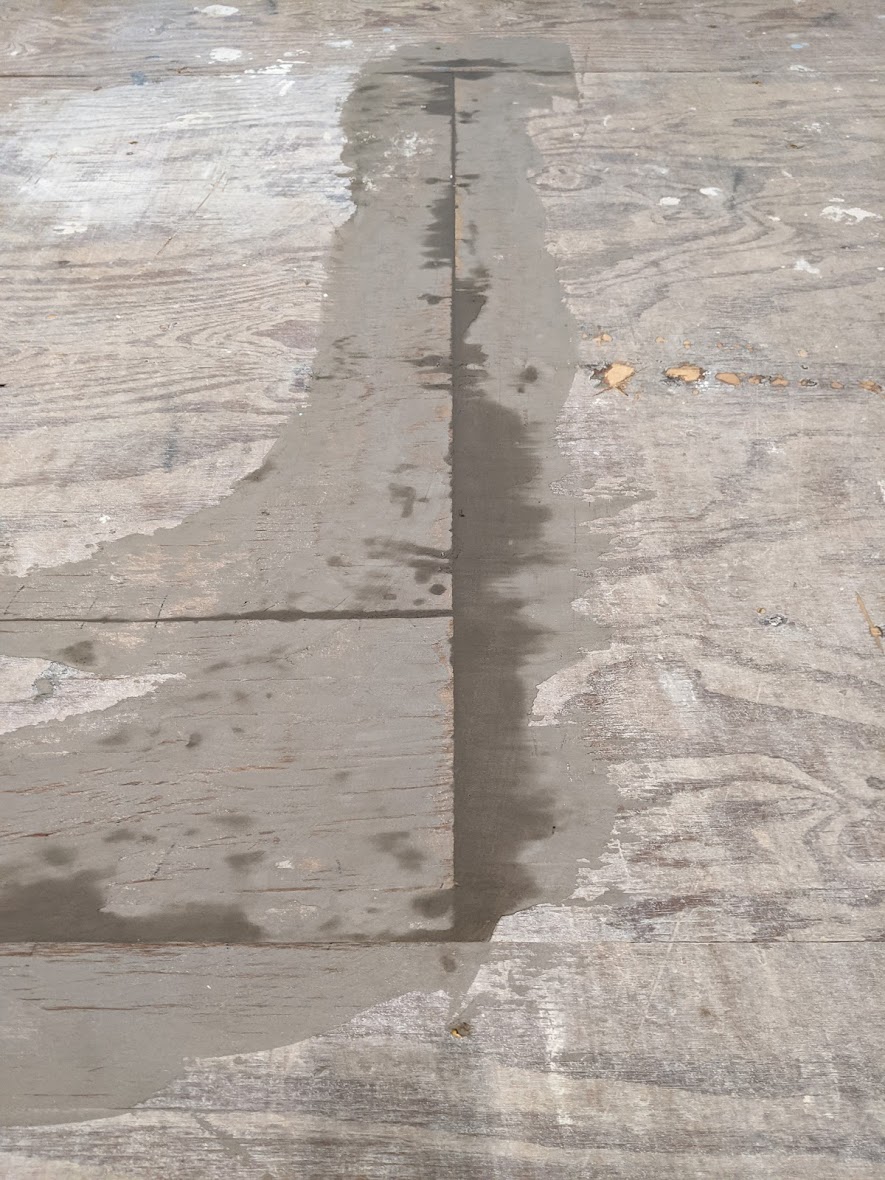
Writing some notes to my future self so I remember these tricks. Tools/materials used: caulk Henry 549 Feather Finish $18 1 gal bucket – I found this was the smallest size that was still convenient for holding my tools water trowel or drywall knife, whichever is biggest margin trowel old plastic takeout container to use…
Read More How to level a wood floor